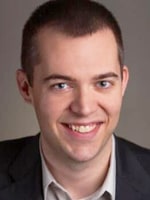
As consumers increasingly turn to e-commerce retail, loss prevention (LP) teams need to step up their fraud-prevention efforts. Every year, “lone wolves” and organized retail crime (ORC) rings globally steal hundreds of millions of dollars by exploiting the anonymity and secrecy associated with online transactions. Only with an advanced analytics solution like prescriptive analytics can retail asset protection (AP) teams successfully uncover and eliminate this fraudulent activity.
Prescriptive analytics is an analytics methodology that combs through data and determines:
- What is happening.
- Why it happened.
- How much it is costing you.
- What to do about it.
- Who should do it.
Here are four common types of e-commerce fraud that retailers have rooted out with prescriptive analytics.
Product Switch-Outs
A typical switch-out scheme involves ORC conspirators ordering a huge quantity of premium items from a retailer’s website, only to return cheap knockoffs instead. The returns most often happen in store, where busy associates are less likely to notice the difference. Thus, the fraudsters receive a full refund for the order while keeping all the premium items to sell for cash.
The key to catching this type of fraud does not necessarily require identifying the returned items as knockoffs. It is about identifying the conspirators’ buying/returning patterns. If a fraudster successfully pulls off a product switch-out scheme once, they will almost certainly do it again and again. This will create a highly suspicious pattern of behavior within your data—consistent purchasing and returning of high-dollar-value orders. Having an analytics solution in which your e-commerce and brick & mortar sales data is joined together allows you to quickly tie customers, addresses, and tenders together to detect these patterns and address them before they become major losses.
Excessive Appeasements
Any retailer who deals in e-commerce will have some sort of customer appeasement process to address orders that are lost in transit or stolen from the customer’s porch. A customer service representative (CSR) will have the ability to re-ship customer orders at no charge, sometimes with additional discount offers or even gift cards to help correct the situation for the understandably upset customer. This practice is critical to maintaining a great customer experience when inevitable issues in the shipping process occur, but it can also carry a major risk of fraud. LP investigators have caught CSRs sending these replacement orders and appeasements to themselves or acquaintances, sometimes as part of a larger ORC ring. The losses to such activity can add up quite fast.
There are two telltale signs of appeasement fraud—suspicious addresses and suspicious frequency of appeasement. The former approach involves looking at the destinations of appeasement orders. Suspicious addresses include:
- Current/former employees’ addresses
- Addresses to which multiple appeasement orders have shipped, sometimes resulting in more appeasements than actual spend
- Addresses that do not match the purchaser’s address on file (the purchaser may be sending the appeasements to an ORC fence location or collaborator)
- Addresses near company call centers or warehouses
Appeasements headed to any of the above destinations should be carefully scrutinized.
The frequency approach to detecting suspicious appeasements begins with using machine learning to benchmark normal appeasement behavior across all CSRs. From there, the right solution, like prescriptive analytics, can easily identify CSRs whose behavior constitutes an anomaly and flag them for follow-up.
The frequency approach helped one specialty retailer uncover an ORC ring fraudulently appeasing orders and sending them to conspirators across their state. Sensing an opportunity, the ORC team enlisted additional individuals to apply for positions at the retailer’s call center, meaning that more conspirators were being hired every week. Thanks to the insights of its prescriptive analytics solution, the retailer was able to act quickly, break up the ORC ring, and eliminate what would have been $50,000 a month in losses.
Payment Card Fraud
One of the most often-seen fraud schemes in e-commerce is card-not-present fraud, in which thieves use stolen cards for fraudulent purchases online, taking advantage of the lack of a cashier to verify the physical card. Fraudulent online purchases impact retailers in many more ways than just lost merchandise: negative brand image for customers who experience fraudulent use of their cards, a time-consuming and costly chargeback process, and loss of shipping and processing fees, to name a few.
To avoid the costly consequences of fraudulent online orders, a key strategy is to look to your data and stop the orders before they reach their destination. A good prescriptive analytics solution will analyze order destinations and flag any addresses with multiple high-value orders enroute simultaneously. This behavior is a strong indicator that the customer is using multiple stolen credit cards to place the orders.
Recently, a fashion retailer used this logic to identify 93 orders destined for a single address in Florida. Thanks to its prescriptive analytics solution’s timely alert, the retailer’s AP team quickly confirmed all 93 were purchased on stolen credit cards and intercepted the shipment. Nearly $10,000 worth of merchandise was returned to the warehouse, and the retailer avoided any losses from this case.
Loyalty Promotion Abuse
To incentivize shoppers for their patronage, many retailers offer e-commerce customers a welcome offer to entice them to sign up, often a discount. But some customers go a bit further by trying to apply multiple discounts (with the help of various false aliases) to a single order – a practice known as “stacking.” Loopholes that allow discount abuse are not unheard of in retail e-commerce operations, and fraudsters will quickly pounce on these opportunities. Some will even create software to automate the “dirty work” of repeatedly adding accounts, vastly increasing the loss impact to the retailer.
Many retailers use prescriptive analytics to identify and eliminate this type of fraud. One especially successful methodology involves flagging e-commerce orders with high discount activity. You can use machine learning to identify orders with anomalous discounts, making it easy to see any suspicious activity, including potential stacking. For added precision, some retailers have configured their solutions to combine this anomaly detection approach with the address-based frequency approach mentioned above to identify specific addresses with frequent discount anomalies. What’s the logic? Fraudsters can easily change the name, phone number, or e-mail address on their orders in attempt to cover their tracks. But if they actually want to receive the product, they’ll need to use a real address – pointing investigators straight to the culprit.
For more information on using prescriptive analytics to stamp out e-commerce fraud, visit the Zebra Analytics website.