Without consciously realizing it, and certainly without having made a conscious decision to pursue it, we as a society find ourselves living in a time when nearly every public thing we do, every decision we make, is recorded. Everywhere we drive, everything we buy without cash, the names of people we talk with, most of what we read—all is being recorded somewhere. Ten years ago this state of affairs would have seemed some fringe conspiracy. But we as individuals have just sort of gone along with it in the vague hope that somebody on the other end is being responsible with the information we either volunteer or have no choice in giving up.
The loss prevention industry finds itself in a position of being one of those “somebodies” on the other end. By nature a conservative, risk-averse beast, the LP industry is well suited to the task of playing steward to this enormously valuable, enormously risky set of big data. And when used properly, LP is also well suited to the role of leader when it comes to guiding other retail segments toward best practices and responsible use.
Analytics is the side of big data that doesn’t have to worry as much about risks. Analytics plays with the data, extracting the patterns and putting them to useful ends. But guarding the data—cyber security—is outside the scope of analytics. Nevertheless, it’s important to keep in mind during any discussion of analytics that all of the data comes from somewhere and could have wide-ranging, possibly calamitous effects if not well protected.
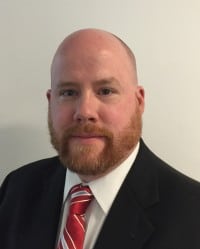
The modern data-focused trajectory of retail has accelerated its own pace of change and with it the rate at which a response is necessary in order for a retailer to remain competitive. “The traditional LP person is focused on tangible things in brick and mortar,” said Tom Meehan, CFI, Bloomingdale’s corporate manager of data, systems, and central investigations. “But today, by the time your loss is tangible, it’s already out of control.”
With the ongoing shift from conventional channels to multichannel, omni-channel, and now unified commerce, staying on top of the data—data that’s coming in from the many disparate avenues of the business—is essential to serving the multitude of platforms that consumers have come to use. “If we follow suit with the industry, we’re going to need to utilize data more to be able to make better business decisions in real time, especially given the omni-channel environment,” said Meehan. “If I didn’t utilize data, I wouldn’t be able to make business decisions. Behavior analytics is, in my opinion, the key to success in our future. I’m a big proponent of focusing on deviant behavior to identify anomalies. It’s looking at the behavior that’s driving the data. What our data guy says is, ‘There’s a lot of noise, but it’s all we have.’”
How Analytics Works
Using computers to search for patterns is all analytics really is. When an LP professional on the floor in a brick-and-mortar store notices certain tells common to bad actors in their stores, or gains a sixth sense when it comes to intuiting an investigation, he is taking inputs of data from his eyes and his ears and, consciously as well as subconsciously, finding patterns in that data. Analytics does the same thing, just using computers instead of the human brain and using hundreds or thousands of data feeds from all across the retail organization instead of human eyes and human ears.
Analytics has gained a certain mystique, surrounded by esoteric terminology like “Markov chain Monte Carlo sampling” or “traversing million-dimensional Bayesian solution space.” And, true, the actual methods behind the approach—what goes on under the hood—is something that requires an advanced understanding of mathematics and statistical methods to really get. But what analytics does is quite simple. What analytics does is automatically, and in real time, look at the cornucopia of data streams available and search for patterns that have an impact on profit.
It supports the eyes and ears of an LP floor team member or investigator with computer files: point-of-sale (POS) feeds, employee time clocks, direct-store-delivery (DSD) manifests, inventories, and other kinds of data central to the running of the business. It can also use less obvious data sources. Video analytics can pull out information like average group size of customers entering the store or heat maps of which aisles or displays customers spend the most time around. Floorplans can be overlaid with all sorts of information pertaining to the within-store geographic centers of profit and loss. Even social media can be used. Crawling Twitter and Facebook posts looking for mentions of the company or of key brands can be used to predict when spikes of sales or loss occur and help illuminate why they occur.
These data are usually available as time series, that is, as data points connected to a timeline. So by lining up the timelines of different kinds of data beside the timelines of profitability, you can see which variables impact shrink as well as which don’t matter as much. Analytics just takes this concept to an extreme of sophistication, comparing thousands of variables, seeing if co-variance between a group of variables can explain profitability fluctuations if a single variable cannot, and searching for patterns that might not be immediately apparent, like if an indicator from six months ago is impacting shrink today. Watching these leading indicators closely can help ensure that the day six months from now where the loss would have occurred never actually comes. Analytics enables the business to act predictively and proactively rather than descriptively and reactively.
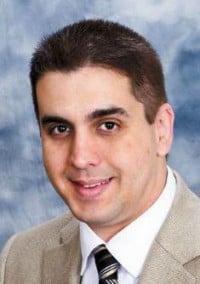
Some indicators may not be direct in their influence on shrink. “We find that high cash over and short activity points to high shrink,” said Johnny Custer, LPC, CFI, director of analytics at Sears, “not necessarily because of direct effects, but because it shows there’s not attention to detail. And if there’s not attention to detail at the register, then there’s not going to be attention to detail for the more complicated things.” In such cases, addressing that one issue won’t fix the shrink problem since the root cause is being ignored. But they are still valuable for the information they provide. “Look at the data points found throughout your enterprise, such as POS, inventory management, and others to see which ones truly have the highest impact on shrink and profit erosion,” said Custer. “Sometimes things like an employee morale test can act sort of like a profitability mood ring. In instances like that where you can isolate and measure some of these more ‘obscure’ data points, it can show you specifically where to focus your efforts.”
When constructing an analytical model, you’re trying to isolate which variables have the most explanatory power when it comes to the factors you’re interested in—profit and loss. But these things change over time, as do the contributing factors. Furthermore, the algorithmic methods and the software tools used are continually improving. What this means is that model building in analytics is not a one-time thing. It’s not like constructing a building; it’s more like the inventory on the shelves inside the building. You’re constantly adding things and taking things away to see what’s most successful.
“One of the main pieces that is important to communicate is that you’re building a predictive model,” said Custer, “It’s a growing, organic report. We’re always adding to it, taking things away, and modifying. What’s important in December might not be important in August. After a few years, when your loss has stabilized, it becomes as much or more about sustaining those results.”
Teaching a New Dog Old Tricks
As the LP function continues to morph into something more integrated with the digital world and the vast and varied streams of data associated with that world, the skillset required of LP professionals also changes. “In the past,” said Meehan, “we’ve always taken LP people and tried to make them analysts. We’d take a guy who was great in the field and put him with a guy who was great at Excel. I’ve found that it’s a lot harder to teach a field LP
guy statistics or advanced mathematics than it is to take a stats guy and teach him how we catch bad guys.”
Each organization will find a different balance between training field LP professionals in analytics and training analysts in fieldwork. “The older generation is very much about LP fundamentals—investigations, catching bad guys, being safety-minded,” said Custer. “Then we have a very new crop of people who are 100 percent data-centric. We have to meet in the middle. I worry that if we go too far to the analytics side, then we lose what we’re really good at. There’s always going to be a need for that ‘gut feeling,’ a need for good investigations. I worry what happens when the new crop of LP personnel are only about analytics. I think anyone entering the field, whether straight from school with a statistics degree or coming from law enforcement or military, or from elsewhere in retail, they need the fundamental, foundational LP view. And they also need to get a comprehensive understanding of the company as a whole, not just catching bad guys.”
The opportunity now also exists for enterprising people either entering the profession or already on the LP path to aim toward exposure and training in as many facets of the job as possible, knowing the role that analytics and big data will be playing in the future as well as the importance of the stock-in-trade in the field. Likewise, the opportunity exists for forward-looking companies to train the next generation of industry leaders holistically, creating opportunities for employees to have exposure to the role through a variety of lenses. Firsthand experience on each side could strengthen the position of the employee in all roles they perform, setting up the organization for future success.
Selling to Operators
Having boatloads of data is all well and good, but it’s useless unless it’s translated into changing the way the business actually functions. And a big part of taking the patterns unearthed by analytics methods into the real world starts with convincing operators, merchandisers, and the like that the data is worth paying attention to.
But it takes more than just showing that you’ve discovered real, significant patterns, Custer pointed out, “It’s a hard transition for an LP program to go from 100 percent traditional LP by immediately shifting 180 degrees and then burying them in data. The key is not just creating and distributing data, but also having strong reports that are digestible and actionable. Unless you give them information they can actually act on, there’s not much they can do about it. They want to see and understand the direct shrink and margin impact, as well as exactly what they can do to influence the numbers.
“Sales reducing activities (SRAs) are one group of actionable leading indicators that the field works with every day. SRAs are any occurrence within a transaction that subtracts from the full value of a sale, such as coupons, voids, and price overrides. A large percentage of investigators still only look at the theft and fraud aspect of SRAs. What happens when I line up those high-ranking SRA stores and draw a direct correlation to shrink and margin erosion in 94 to 97 percent of your stores? This should raise a number of eyebrows. Certainly we can write off a good portion of SRAs to customer service, an expected and sometimes necessary part of driving sales. However, a significant number of SRAs are due to fraud, operational deficiency, poor training, or even flat-out laziness. Because of these strong correlations, I can leverage SRA and other leading indicators to produce an actionable report that tells me store 1234 has a 70 percent chance of hitting the wall six to eight months from now. Using the data as an educational tool, showing the math—that’s how you build credibility in your program. That’s how you get people to listen to you. You can’t argue with math,” explained Custer.
It’s also how the LP role becomes a more central, more valued part of the business. “If we can influence our partners to see why these numbers are so important,” said Custer, “We can sit down and have productive conversations with our store partners and educate them on whether and why we think their issue is theft, a training issue because of one thing, or an operational breakdown because of something else. We can then provide informed recommendations. Now, all of a sudden LP gets a much more important seat at the table. We’re now talking about driving sales, improved customer service, better gross margin; not just minimizing the bad stuff.”
Along the same lines, analytics can serve as a bridge into other parts of the business. Since other teams in the business are also realizing the value of analytics, they are also collecting data and building models to further their own goals. Reaching out to those personnel elsewhere in the business who are involved in similar tasks could result in mutually beneficial data sharing and workload reductions through fresh perspectives and redundancy elimination.
“It’s about getting the right people on the phone,” said Meehan. “Data is a very unique skillset. I hired a guy with a master’s degree in statistics, and what he did right when he got here was get me involved with people I would never have known about in other areas of the business because I don’t speak their language. LP has always had good data. We have data they don’t have, and they have data we don’t have. We have different strengths and focuses, but those can be complementary. So if you go into the meeting with the mindset that we have a common goal of profitability, when you’re speaking the same language and have the same goals, you’re going to get good results. For example, marketing teams make look-alike models to replicate good customers. How many times have you sat down with your marketing team and said, ‘Let’s look at what a bad customer looks like’? The math is exactly the same. All you have to do is reverse engineer it. The opposite of good is bad.”
Big Data, Big Risk
With the power of analytics comes responsibility. Collecting and storing vast amounts of data has come back to bite thousands of organizations over the past several years. And many were truly massive breaches in national or global organizations—Target, Home Depot, the Department of Justice. Even the Department of Defense has had its system breached and massive amounts of personal data stolen (and in their case also highly sensitive and highly valuable classified documents). It’s bad enough when data thieves take credit cards. While there are systems in place to deactivate stolen card numbers and reissue cards to the rightful cardholders, consumers still suffer, and the damage done to a brand can be enormous. But when more than credit card data is stolen—especially personal data—the damage to consumers and the subsequent damage to a company can be downright catastrophic.
Identity theft is nothing new. What is new is the scale of exposure. A moderately sized national retailer may store highly personal identifying information on millions of customers. And the kinds of information being retained are unprecedented. What havoc could thieves wreak with data about millions of Americans’ brand preferences and buying habits? Nobody may quite be sure yet, but if history has taught us anything, it’s that it would be foolishly reckless to assume that such data is useless to attackers and would present no risk. Before computers, nobody (except a few sci-fi authors) imagined that computer viruses would be a risk; the risks of the future are unthinkable until the systems that enable them are actually built and used for a time. New systems create alongside them entirely novel risks and avenues of attack.
The damage potential of highly public revelations of data theft should ensure any company embarking on large-scale data collection does so in the most careful, deliberate, systematic way possible, taking into account all possible vulnerabilities and designing redundant systems to protect against any imaginable attack—and ideally to protect against unimaginable attacks as well. Clearly, not all companies have approached data collection so responsibly. Each LP team member is responsible for doing their own part—even if it’s as small as practicing good password habits—in ensuring that their own organizations’ systems are secure. But even the most diligent team can be affected if other companies are slack. The risks of consumer pushback, overburdening legislation, and social movement away from free, careless data sharing exist if any one member of the corporate community suffers an attack. Each individual company’s failure reflects on the whole, as consumers think, “If this can happen to company X, why not to company Y?”
And so the potential for a sort of tragedy of the commons exists. It is in each organization’s best interests to ensure that other companies, even competitors, are using best practices to ensure their customers’ data is secure. Industry meetings and taskforces exist to help further this end, but data security is one area where a proactive approach is absolutely essential.
And solutions do exist. Protecting data is not impossible. It is the job of the system architects, the cyber security team, and IT networking much more than the analytics team. But analytics is still generating and storing important new data and needs to make sure it is playing its part. “Cyber security is independent and has to be a part of every single systems initiative you have,” said Meehan. “You have to keep it separate from business intelligence and big data. The truth is, we’ve always had the most amount of personal data. It’s not because we’ve wanted to, but because we’ve had to. Without it, we can’t effectively do our job. But there are lots of things we can do on our end to build systems to protect the data. It’s important that everybody in the company knows what we have, where we got it, and what we’re doing with it. So we need to make sure to have the same checks and balances in place to make sure to protect the company’s profits for our own sake and our customers’ sake.”
Many companies have built systems that mask personally identifying information. By looking at the same data, investigations can be pursued and cases built without looking at personal information. Instead of watching “Alice Susan Smith, birthdate 08/15/1988,” an investigator can watch “Case 385825” or “Person Turquoise.” “I don’t need to know it’s you right away,” explained Meehan. “I don’t need to know it’s you until I’ve built a case. You can build a system that’s 100 percent masked and protected and still identify the same behaviors. I’d still say we’re in infancy. We’re not ever looking at details until I have built a case and am ready to apprehend.”
The point isn’t to mask a suspect’s identity from investigators. The investigator isn’t the person customer data needs to be protected from. The point is that if a system has been put into place that makes all customer data pre-anonymized, with proper encryption and access protocols, then if that system or database was stolen, a thief would have information about “Customer 959544” instead of “Alice Susan Smith.” Actually, if properly implemented, instead of “Alice Susan Smith, birthdate 08/15/1988, makeup brand preference X, bread brand preference Y,” the thief would come away with a bunch of hashed, encrypted gobbledygook like “JFDfj#ajd$f7t02jfjjfa09fa&F.”
Piecing It Together
One recurring issue when talking about analytics is that everybody talks about it a bit differently. According to Meehan, this is a reflection of the fact that everyone in the LP industry is in a different place when it comes to analytics. “Some folks have one guy on Excel, while others have whole teams of people dedicated to analytics. The industry is using much more sophisticated tools today compared to three years ago, but it’s important to realize the limitations of what you’re doing. In the LP world I rarely talk to anyone who is doing anything more than linear regression and basic modelling. That kind of thing might even be a detriment because what you’re getting from it is not what you think you’re getting.”
Each organization is going to piece together its own solution in its own way, and there’s nothing wrong with that. But building an analytics solution is a process; there are no plug-and-play, turnkey solutions like there are in other technological domains. “There are folks out there looking for that secret sauce, one-stop solution,” said Meehan, “but that really doesn’t exist.”
Advances in the fields of analytics happen at universities, in the pages of statistics and machine-learning journals, and on the project pages of software development groups. There is already enormous variety in methods and approaches, but as LP more fully embraces data analytics as a core competency, more attention will be paid to breakthroughs in the field. New, better ways of doing things will be adopted. Enterprising teams who develop methods in-house may even contribute to the field by publishing their approaches. All in all, the data side of LP stands to gain from opening a dialogue with its analogues in academia. “If we’re not experts, if we don’t have experts in the industry, then we need to lean on the experts,” said Meehan, “and there’s nothing wrong with that.”
But continuing to emphasize the foundational approaches to loss prevention will be more important than ever, as the scope of the LP role within an organization broadens and blurs. Especially important will be training the new computer- and statistics-focused hires on traditional LP skills and on how the business works as a whole, preemptively addressing potential issues of scope myopia. Finally, making sure to protect customers’ personal data should be an omnipresent imperative for anyone working in the field. There is huge potential risk if data is mishandled, and the ways that analytics is modifying these risks hasn’t been well explored. Once again there is a prime opportunity for the LP industry to take a leadership role in exploring these risks, reaching out across business lines, and mitigating them. It’s a new path, and it’s dark up ahead, so we should walk carefully.