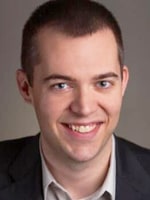
Most of us in loss prevention (LP) have heard of the “banana scheme,” the self-checkout fraud where customers enter the PLU code for bananas while weighing up pricier items instead. We’ve also heard how challenging it is to catch and eliminate, especially if the suspect claims it was just a mistake. But have you tried identifying and eliminating this self-checkout scheme with data?
The right analytics solution, like prescriptive analytics, can flag telltale data behaviors that both reveal the banana scheme and, in some cases, even hint to the perpetrator’s intent (malicious or non-malicious). Here are three of those data behaviors.
Multiple Line Items
When bananas appear on multiple lines in an order, it’s a pretty good indication that something fraudulent is going on. Think about it—we’ve all bought multiple bunches of bananas at one point in time, but how do we typically ring them up? Most customers will place all their bananas on the scale and weigh them all together. Someone who is trying to pull off the banana scheme, however, might weigh the items they want to steal one at a time to preserve the appearance of a legitimate transaction.
An analytics solution can be configured to alert the LP team when these suspicious transactions happen repeatedly for certain customers, or when certain self-checkout (SCO) attendants happen to be working, in order to identify the fraud and allow the team to take action to prevent future loss.
Excessive Weight
Have you ever bought 15 pounds of bananas in one go? Unless you happen to work for your local zoo, probably not. This rare occurrence is yet another data anomaly that you can use to identify the banana scheme. Any abnormally large purchase can be flagged for an audit by your analytics solution. Chances are the customer is weighing a jug of laundry detergent, a full beef roast, or something similar at the price of bananas.
The exact definition of “excess” can vary by store or retailer, making analytics an invaluable tool in this methodology. The right solution will calculate the average quantity of bananas purchased across all customers, making it easy to spot any outliers. Additionally, as the LP team addresses the theft concerns and the averages decrease, the tool will follow along and adjust its thresholds to account for the change in the data.
Frequency of Purchase
When buying bananas, the typical grocery shopper buys a sizable quantity early in the week, sometimes with another supplemental purchase towards the middle of the week. When a customer appears to be buying large quantities of bananas every other day, a red flag automatically goes up. It may sound like a stretch, but it’s a great indicator of the banana scheme. The customer in question likely got carried away with their first few attempts and is now leveraging the scheme every time they go to the store.
Though we’ve discussed the banana scheme in detail here, it’s important to note that a properly configured analytics solution can identify many other anomalies at SCO as well. From age check non-compliance to repeatedly scanning a low-price barcode hidden in a thief’s palm, nearly all SCO issues leave a data trail, and prescriptive analytics is just the solution to identify and address them.
To learn more about using prescriptive analytics to stop self-checkout fraud, see Zebra’s new e-book, “4 Simple Data Behaviors that Give Away Self-Checkout Fraud.”