In 2017, the first book on analytics for loss prevention was released, Essentials of Modeling and Analytics: Retail Risk Management and Asset Protection. The book explores the relationship between criminology theory, analytics and loss prevention.
In a series of articles for LP Magazine, the authors highlight a few thought-provoking topics included in the book. This post focuses on how criminology theory and analytics play a role in loss prevention.
At the foundation of loss prevention is criminological theory. Criminological theories provide us with a framework to assess loss prevention situations and solve problems in a structured way.
This post is designed to provide a theoretical context for understanding the psychological and social forces that drive people to become criminals, how criminogenic opportunities can be reduced, as well as where analytics fits into loss prevention. See Figure 1 for a loss prevention strategy using theory and analytics.
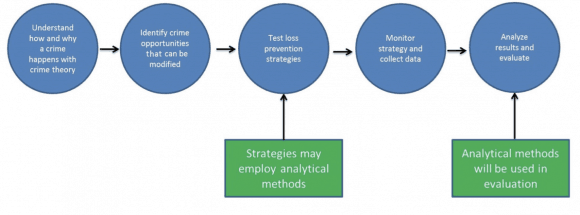
Why does crime occur more in some places than others? The design of physical space is important in understanding criminal events. Situational crime prevention is a preemptive approach that consists of techniques, derived from criminology theories, used to impede the commission of crimes by making the crime more difficult and/or less profitable.
Situational crime prevention is predicated on the routine activity theory and asserts the convergence of three key elements needed for a crime to occur: 1) a likely offender, 2) a suitable target, and 3) the absence of a capable guardian (Felson, 2002).
Thus, larceny is possible when a motivated offender and a suitable target converge in space and time, in the absence of a capable guardian (Clarke, 1997; Cohen & Felson, 1979; Felson, 2006). Implementing guardianship is therefore a necessary condition to prevent loss (see Figure 2). Guardianship is vital in a proactive prevention strategy and can be achieved by various methods, such as implementing audiovisual devices (i.e., enhanced public view monitors or CCTVs).
Data analytics is also an integral part of crime prevention techniques. If, according to the routine activity theory, guardianship or controllers are necessary to reduce the opportunity associated with crime, then which techniques are effective? For how long are they effective? What is the cost-benefit relationship for these techniques? Data analyt¬ics help loss prevention teams answer these questions.
Randomly using experimental and control groups of stores—and tracking key metrics, such as shrink, sales, employee turnover, line voids, return rates, and other outcomes, in before-during-after analyses—loss prevention professionals can accurately calculate a loss prevention solution’s (e.g., a surveillance system) return on investment.
Situational Crime Prevention
Situational crime prevention is derived from the notion that people make choices rationally and include both contextual factors and a cost-benefit analysis (Cornish & Clarke, 2003; Farrington et al., 1993). Furthermore, situational crime prevention depends on the idea that an offender is rational, and will gauge the environmental risks involved in committing a crime (Clarke, 1997).
Situational crime prevention employs measures that are directed at retail theft and succeed by means of manipulation of the environment to: 1) increase the perceived effort of committing the crime (e.g., target hardening and access control), 2) increase the risks of committing the crime (e.g., extend guardianship), 3) reduce the rewards from committing the crime (i.e., decreasing the benefit), 4) reduce the opportunities for crime, and 5) remove excuses for committing the crime (Clarke, 1997; Cornish & Clarke, 2003; Sutton et al., 2008).
Situational crime prevention is based on classic research methodology: 1) collect data regarding the nature of a specific type of crime, 2) analyze the situational conditions that facilitate this crime, 3) study possible methods to block opportunities for crime, 4) implement the most effective, feasible, and economic measures, and 5) monitor the results and propagate the knowledge.
Many of these steps are heavily dependent on data sciences techniques. To maximize their benefits, data must be collected using fair, consistent, and accurate methods. Outside situational factors are best controlled through randomization during the selection of cases. Analyzing possible measures to block crime opportunities involves the use of analytical methods ranging from simple pre-post testing to artificial intelligence algorithms and machine-learning models.
Rational Choice Theory
Rational choice theory assesses the decision-making processes that may lead an offender to choose to become involved retail theft (Clarke, 2005). Its main supposition is that offending is a purposive behavior intended to benefit the offender.
Offender behavior is guided by hedonistic values; potential offenders seek pleasure and avoid pain. Retail crime can be prevented by increasing an offender’s subjective probability of being caught. Thus, an offender’s behavior can be manipulated by altering environmental cues. With this knowledge in place, successful loss prevention can be achieved if the costs are made higher than the benefits of committing a crime (e.g., enhancing protection, target hardening, and surveillance).
In 1999, Clarke posited the CRAVED model, which was aimed at the theft of hot products. According to Petrossian and Clarke (2014), the acronym CRAVED is heuristic and enables us to understand what makes targets especially attractive to potential offenders. CRAVED incorporates six properties: 1) Concealable – Items that can be hidden are more vulnerable to shoplifters, 2) Removable – Items that are easy to remove, such as beauty aids, medicine, cigarettes, and alcohol, 3) Available – Items that are accessible and easy to attain, 4) Valuable – Offenders will generally choose the more valuable items, particularly when they want to resell, 5) Enjoyable – Hot products are likely to be enjoyable, for example: liquor, tobacco, and games, and 6) Disposable – Offenders tend to select items that are easy to resell, this explains why batteries and disposable razors are among the most stolen items.
Crime Prevention Tools and Principles
Many analytical tools can be used to study and deter crime. Randomized controlled trials, for example, can be used to study physical prevention tools in the store. Hayes and Downs (2011) conducted a randomized controlled trial of three situational crime prevention tools: in-aisle CCTV public-view monitors, in-aisle CCTV domes, and protective keepers to assess their effectiveness in deterring theft of a hot product (i.e., razor blade cartridges).
The study found that all three prevention measures significantly reduced shrink compared with control stores (Hayes & Downs, 2011). The tested treatments were designed to reflect the situational crime prevention framework, as they were each designed to prevent crime events by making theft more difficult or risky for offenders.
Modeling and advanced machine-learning techniques can be used to monitor and detect odd consumer and employee behavior. Outlier detection is one method that can be used to determine internal fraud. For example, an employee may use line voids to “give” the customer (who is a friend or family member) merchandise without paying for it. To the retailer, the transaction appears legitimate, but when inventory is counted, the item is short.
When conducting outlier detection, the analyst must determine the expected value for the metric in question. For example, what is the normal rate of line voids in a store? To determine the expected rate, the analyst will want to compare the employee to like employees, stores to like stores, and account for seasonality and product type. Once the expected value is determined, outlier analysis reveals which records are far enough from the norm to arouse suspicion of sweet-hearting.
Loss prevention can also be implemented using the SARA pro¬cess—scanning, analysis, response, and assessment (see Figure 3). Once crime patterns are scanned, identified, and narrowly defined (e.g., theft of handbags), the loss prevention team can analyze the issue to understand both its characteristics and causes. Based on this analysis, the loss prevention team can develop responses to prevent future theft (e.g., benefit denial or surveillance).
Crime prevention through environmental design (CPTED) is based on reducing crime by manipulating the design of the built environment (Cozens, 2008; Crowe, 2000; Reynald, 2011). The main purpose of CPTED is to harden the targets for a potential offender.
In conclusion, we have developed a set of principles to assist in loss prevention. These principles are the culmination of studying the many components of loss prevention. The principles are built on three key elements: prevent, respond, and solve. These are not all-inclusive, but will be valuable for any team’s efforts to prevent loss.
Ten Principles—Prevent, Respond, and Solve
1. Build an analytical crime prevention team—join forces—prevent.
2. Develop a crime prevention plan—prevent.
3. Educate and train employees—prevent.
4. Know and protect theft targets—respond.
5. Change the environment—don’t be vulnerable—decrease opportunity—respond.
6. Collect good intelligence and use reliable data—garbage in, garbage out—solve.
7. Embrace technology—facial identification, target profiling, nanotechnology—solve.
8. Predictive analytics—examine patterns—solve.
9. Analyze techniques—evaluate return on investment—solve.
10. Adapt, iterate, and improve—prevent, respond, and solve.
EDITOR’S NOTE: This post has been excerpted/adapted from the authors’ text, Essentials of Modeling and Analytics: Retail Risk Management and Asset Protection. Learn more.
References
Clarke, R.V. (1997). Situational Crime Prevention: Successful Case Studies. 2nd ed. Albany, NY: Harrow and Heston.
Clarke, R.V. (1999). Hot Products: Understanding, Anticipating, and Reducing Demand for Stolen Goods. Police Research Series, Paper 112. London, UK: UK Home Office.
Clarke, R.V. (2005). Seven misconceptions of situational crime prevention. In: N. Tilley (ed.). Handbook of Crime Prevention and Public Safety. Portland, OR: Willan Publishing.
Cohen, L.E. & Felson, M. (1979). Social change and crime rate trends: a routine activity approach. American Sociological Review. 44:588–608.
Cornish, D. & Clarke, R.V. (1986). Introduction. In: D. Cornish & R.V. Clarke (eds.). The Reasoning Criminal. pp. 1–16. New York, NY: Springer-Verlag.
Cornish, D.B. & Clarke, R.V. (2003). Opportunities, precipitators and criminal deci¬sions: a reply to Wortley’s critique of situational crime prevention. In: M. Smith & D.B. Cornish (eds.). Theory for Situational Crime Prevention: Crime Prevention Studies. vol. 16. pp. 41–96. Monsey, NY: Criminal Justice Press.
Cozens, P. (2008). Crime prevention through environmental design. In: R. Wortley and L. Mazerolle (eds.). Environmental Criminology and Crime Analysis. Devon, UK: Willan Publishing.
Crowe, T. (2000). Crime Prevention Through Environmental Design: Applications of Architectural Design and Space Management Concepts. 2nd ed. Oxford, UK: Butterworth-Heinemann.
Farrington, D.P., Bowen, S., Buckle, A., Burns-Howell, T., Burrows, J., & Speed, M. (1993). An experiment on the prevention of shoplifting. In: R.V. Clarke (ed.) Crime Prevention Studies. vol. 1. pp. 93–119. Monsey, NY: Willow Tree Press.
Felson, M. (2002). Crime and Everyday Life. 3rd ed. Thousand Oaks, CA: Sage Publications.
Felson, M. (2006). Crime and Nature. Thousand Oaks, CA: Sage Publications.
Hayes, R. & Downs, D.M. (2011). Controlling retail theft with CCTV domes, CCTV public view monitors, and protective containers: a randomized control trial. Security Journal. 24(3): 237–250.
Petrossian, G.A. & Clarke, R.V. (2014). Explaining and controlling illegal commercial fishing: an application of the CRAVED theft model. British Journal of Criminology. 54:73–90.
Reynald, D.M. (2011). Factors associated with the guardianship of places: assessing the relative importance of the spatio-physical and socio-demographic contexts in generating opportunities for capable guardianship. Journal of Research in Crime and Delinquency. 48:110–142.
Sutton, A., Cherney, A., & White, R. (2008). Crime Prevention: Principles, Perspectives and Practices. Cambridge, UK: Cambridge University Press.
This post was originally published in 2017 and was updated October 23, 2018.