In the 2002 futuristic movie Minority Report, John Anderton, played by Tom Cruise, commands a unit called Precrime. It is the role of this specialized law enforcement group to arrest people who are about to commit an offense with the information provided by Precogs—humans with the unique ability to predict the future. When Anderton questions an anomaly he has discovered in one of the Precogs, he instantly becomes a fugitive on the run. Scenes of Cruise’s character attempting to evade his former crime fighting unit illustrate the near impossibility of avoiding arrest as citizens are constantly under camera surveillance.
Although Minority Report features the quintessential battle between good and evil, making for box office success, the reality is that having the ability to predict whether or not a crime will take place is not science fiction. Artificial Intelligence (AI) capabilities, as part of security infrastructure, are available now even if they do not come with the aforementioned Precogs. The limitations of prediction, however, are significant and ultimately require humans to decide whether or not an individual will commit a crime and what to do with that information. Therein lies a philosophical, ethical, and moral debate—if you know that an offender is on your premises because of artificial intelligence-backed data garnered through your security cameras, does that mean that they are going to commit a crime, and if so, what can you do to stop them?
Identifying Criminals
Criminologists have been building predictive models for decades. I worked on early versions of these models as a university student from 1989-1993. Then, our work focused on quantifying crime scenes by giving certain aspects or features of the scene a numerical value from one to ten. We then quantified the records of known offenders. Our model matched the offender with features from previous crimes and provided a predictive analysis of the likelihood that they would commit an offense in the same category as the current crime scene. This naturally allowed law enforcement officers to narrow their search. The results were so effective that federal agencies later used the model.
After university, I forayed into federal law enforcement. There, the techniques we used were more subjective. We were not using computers. We used gut instinct based on a combination of skills, observation, and experience. Ultimately, even though our predictions were less data-driven, they did provide a high degree of accuracy. Today, top law enforcement professionals will tell you that although they benefit from advanced technology, gut instinct and experience are required when dealing with the constantly evolving world of crime.
In retail, the rise in theft, violence against employees and customers, social media-driven anarchy in an attempt to gain more likes and followers, and shoppers feeling unsafe in their favorite stores have left asset protection and store security professionals actively seeking technological solutions that will assist in stemming the rising tide of brutality. Questions, however, remain as to the effectiveness of current solutions, implementation costs, the ease of adoption, and how much disruption it will cause to a multitude of stakeholders, from operations to legal teams. Ultimately, will the temporary challenges of adding new technology be worth the time, effort, and energy required?
Retail and AI
Several national retailers are investing heavily in artificial intelligence pilots and production level programs to support their asset protection and store security teams in dealing with the prominent issues caused by organized and disorganized retail crime. From using computer vision to detect if a person is on the ground and then having a real-time alert sent to a security guard or detecting if someone is walking out of a store without paying, technological solutions are providing invaluable data to the growing number of businesses with the foresight to utilize AI.
In the retail sector, artificial intelligence usage continues to rise. Data published by Fortune Business Insights reported that the global AI in the retail segment is expected to grow from $4.84 billion in 2021 to $51.94 billion in 2029. Computer vision dominates almost 40 percent of all applications of any form of AI. The power of computer vision is that it provides insights through numerous existing security cameras at one time, inherently processing significant amounts of sensory data that would be otherwise impossible for one human.
Take a moment to consider the following use case. Imagine that a retailer has 5,000 locations. Each location has 25 security cameras for a total of 125,000. This retailer has a command center where a talented group of people watch the feeds from the cameras as they go by, hoping to identify if something is amiss. The reality is that a team can only monitor so many feeds simultaneously. This is where AI comes in. Models trained to identify real-life scenarios, such as a group of people gathering at the entrance of a store, can immediately put that camera view on the screen. The human then decides to call law enforcement or store security based on what they see from that view or multiple angles of the same incident.
One head of store security for a national retailer informed me that their team often doesn’t realize that an incident has occurred until after law enforcement has left the scene. Furthermore, in some cities where they operate, the store receives a bill when a law enforcement officer is dispatched to an event that wasn’t life-threatening or considered a crime. The AI use case they are considering would have the technology send a real-time notification to their command center when one of their defined incidents is taking place, allowing the team member to help support the employees that are onsite.
Another legitimate use of AI is the previously discussed power of predicting a crime. Although we are far from—and hopefully never get to—John Anderton’s world of sedating humans with psychic abilities, AI can create real-time alerts which can be used predictively. For example, in the gas station-convenience store sector, bladder truck theft can cost a station thousands of dollars and a chain, millions. Bladder trucks are vehicles such as pick-up trucks and vans that have been ‘hollowed out’ to hold substantial amounts of fuel.
After the seemingly normal looking vehicle pulls up to the dispenser, one of the occupants inserts a specialized device which radically decreases the speed at which the point of sale is processing the price and steals hundreds of gallons of gas. The vehicle might be at the dispenser for an hour or more which would seem obvious to a human however employees are not always able to see what is happening outside the store.
A simple predictive AI solution would be to identify when a vehicle has been at a dispenser for longer than a specified period of time and send an alert to an employee who simply shuts down the dispenser. If the vehicle is known to be a bladder truck, the AI can record the license plate number and send an alert when that vehicle is on premises at other locations. This use case is predictive as it makes the assumption that large vehicles parked at dispensers for longer periods of time are potential risks for theft. Attaching the license plate of a known offender serves to increase the likelihood.
With growing problems come many opportunities to pilot AI as a solution. Simply deciding to explore computer vision is a logical first step. Retailers tend to get excited with the prospect of using AI to offload the burden of dealing with the ever-growing weight of increasingly complex challenges. In the last three years alone, there has been a pandemic, supply chain issues, a labor shortage, changing laws allowing for bad actors to walk out of stores with merchandise without repercussion, and an increase in both organized and disorganized retail crime. Data published by the Retail Industry Leaders Association (RILA) illustrated that pre-pandemic retail theft accounted for $68.9 billion, with $125.7 billion in economic impact and over 658,375 lost jobs.
Putting a Pilot in Place
At our recent Loss Prevention Foundation meeting in Charlotte, many retailers expressed deep frustration with what they feel is the post-pandemic wave of organized retail crime (ORC). There were also several discussions about AI pilot programs. The vice president of asset protection for a large retail organization openly stated, “I will pilot anything right now.” That kind of openness and enthusiasm to solve problems with new technology will ultimately shift the landscape and likely bring a semblance of order back to retail.
Technology pilots are rapidly becoming more mainstream in retail. The leadership teams who understand the specifics of how to execute a successful pilot are much more likely to see tangible results. A 2022 Gartner study found that only 54 percent of AI pilots make it to production. This number is up from 8 percent just a few short years ago. In other words, enterprises are gathering more value, improving the internal practices of pilot execution, and setting clear expectations.
As the co-CEO of a production-level computer vision company, I have spoken about executing a successful AI pilot at many conferences this year, including LPRC. By following specific steps, an enterprise is much less likely to waste money and more likely to solve the problems they face using technology. After observing companies who have failed to go from pilot to production and interviewing retailers, there is a clear path to a successful computer vision pilot.
Step 1
The first step is to identify the problems your organization hopes to solve and then weigh them according to priority. It is essential to create a hierarchy of this list and attach real value to each item. Your pilot should seek to solve one to two issues that are having a tangible impact. Having multiple stakeholders from store security, asset protection, operations, human resources, and sales will ultimately help to expedite the process even if there are some frustrating conversations to be had in order to get to unity.
When one division, and one division alone, embarks on a pilot, it is more likely to fail. The key reasons are:
- All production-level AI requires a budget to scale and as many different departments can benefit, it is better to share the budget.
- The ‘last to know’ teams become resentful that technology that could possibly help them was implemented without their knowledge. This makes it nearly impossible to get buy-in to scale the solution.
- The company providing the AI will spend more time ‘selling itself’ to multiple stakeholders at multiple times than deploying the solution. It is a waste of time for both parties.
To ensure a successful pilot, bring everyone to the table, as counterintuitive as it may seem.
Step 2
The second step is to begin vetting independent software vendors (ISVs). Like any solution, there are strong discrepancies between solution providers. Many retailers will go to their own existing network of vendors to get recommendations. Solution integrators are a great resource as they have worked with certain ISVs and can give their honest opinion. Furthermore, server and camera providers also know who is delivering real AI and who just has a PowerPoint and a website.
Qualified ISVs should have experience deploying their solution at scale, be able to provide a customer reference under NDA, be vetted through one or more hardware partners, be at revenue, have appropriate insurance coverage, and be capable of illustrating the validity of their technology. With regard to asset protection and store security, having either an advisor or team member who has experience is extremely beneficial.
Retailers who are uncertain about solution providers will often bring in two to three ISVs and have those companies present to the multiple stakeholders all at once. The reward for the winning ISV is the pilot. It is strongly suggested that these presentations be done in-person whenever possible. You have to be able to look these providers in the eye, spend time with them, and judge their character as hard as you judge their technology.
Step 3
Once you have selected the ISV, your team must define the scope of the pilot if you have not done so already. It is critical to pilot at more than one location to garner the greatest benefits. Single store pilots do not often produce adequate information to make an at-scale deployment decision for a variety of reasons, notwithstanding that very rarely does one store represent your entire organization, environmental and demographic factors could skew data, single store infrastructure might not be scalable, the staff at one store may inadvertently manipulate the data if they are aware of the pilot, and there is no ability to compare store-to-store to discern anomalies in the data.
For large retailers with 1,000 or more locations, 10-30 store pilots are suggested. It is advisable to pick from a selection of poorly performing and high performing stores in a variety of regions. For retailers with a smaller footprint, three to five stores may offer a solid representative sample. Note, more experienced ISVs are not as likely to embark on single store pilots as the cost of implementation is not worth the risk of not deploying at scale. Your ISV is your partner to help solve your problems so ensuring that it is also worth their while builds a solid foundation in the relationship.
Your pilot should last for 60-90 days. If you have an experienced ISV who has already gone to production, you will not be engaging in a proof of concept. The ISV merely illustrates that their existing technology is able to work in your environment. If you have a newer ISV, who is fairly inexperienced, you will want to stretch the pilot out longer to give them time to prove themselves through the phases of integration, deployment, and data validation.
Hardware Considerations
When your ISV presented their solution, they more than likely had a proposed hardware configuration that included camera requirements, an edge device, connectivity, and cloud architecture. Real-time computer vision requires a high level of compute capability and this is served through graphics processing units (GPUs). Although the cost of hardware has come down over the years, the reality is that the more security cameras you are utilizing for analytics and the greater the need for real-time information, the more compute power will be required. At scale, your organization may choose to do a cap-ex model and amortize the entire solution over years which can be extremely cost-effective for your multiple stakeholders.
For your pilot, some ISVs will be able to have the hardware sponsored by a provider. These ISVs have built solid relationships and want to illustrate their capabilities on a solution that they have worked with before. For the hardware provider, it is an opportunity for the retailer to try before they buy. The additional benefit of running your pilot on separate hardware is that it is more secure. The separation allows for the pilot to operate in its own bubble and not interfere with existing systems. If you do not have edge assets, you will be experimenting with new technology. If you have existing edge assets already running AI use cases, the ISV can also prepare an architecture report on how and if they can integrate when the pilot goes to production.
Check-Ins
From a performance perspective, it is important to schedule regular check-ins during the pilot. At these times, your ISV will provide you with updates on the key performance indicators that were set forth in your master services agreement. It is imperative that you do not add additional use cases during the pilot. This can cause a reset and will only serve to frustrate both parties. Focus on the intended goal outcomes that your team set forth during the initial stage of vetting ISV’s. In other words, do not change the rules of the game while the game is being played.
One Last Thing
Lastly, know that computer vision can give you a significant advantage. From solving existing problems to acting as a deterrent, AI enabled solutions are able to give your organization a consequential advantage. The technology can not only empower your existing team, it can be considered one of the team—thus preventing any need for a John Anderton, Precog world.
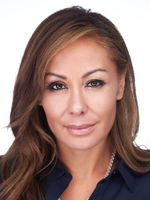
Susan Sly is the co-CEO and co-founder of RadiusAI, an award-winning computer vision company deploying at scale. Susan has appeared on CNN, CNBC, and FOX, and has been quoted in Forbes, MarketWatch, and Venturebeat among others. She has spoken on artificial intelligence and computer vision for MIT, NVIDIA, Lenovo, and others. In addition to being a mom, Susan has dedicated years of her career liberating girls from child trafficking.